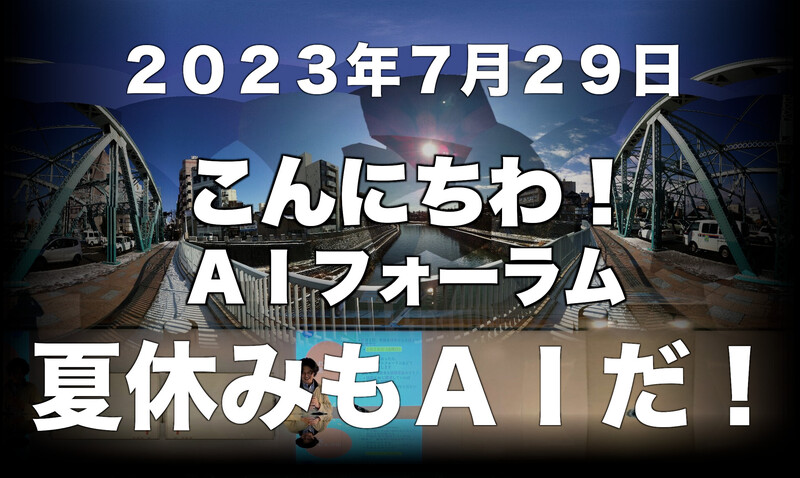
こんにちわ! AI FORUM
2023 年 7 月 29 日 (土曜日)
2023 年 7 月 29 日 (土曜日)
<本日のテーマ>
夏休みもAIだ!
目次
YouTube のアーカイブ・ビデオはこちら
前座
2023 年前半の振り返り
(本題に入る前のウォーミングアップ)
- 今日は7月29日
- 今年ももう半分以上過ぎてしまいました
- 本当なら、先月、ちょうど半分ってことでやるべきネタでしたね
- なぜ今?
- 「こんにちわ! AI FORUM のポッドキャスト」 というものがあります
- 週2回、水曜日と日曜日に、過去のフォーラムのイベントから エピソードを切り出して配信しています
- それが、今週の水曜日(7月26日)シーズン37に入りました
- これが、やっと今年1月のイベントで、
S37E01 (前座)1年の計は1月の ZAF にあり - その1、個人の目標
今年の目標、半分以上、進んでるかな?とおもったから
- 今年の目標は、
今年1月のフォーラム (ZAF-2301) にて
ピアノ
Twitch で頑張ってる!
- KAF-2306 でもまとめました
ポッドキャスト その1
「こんにちわ! AI FORUM のポッドキャスト」
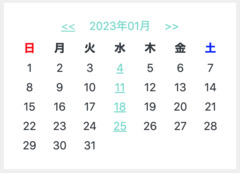
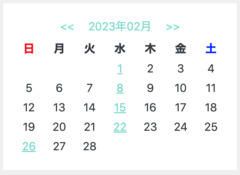
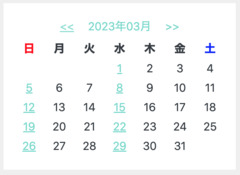
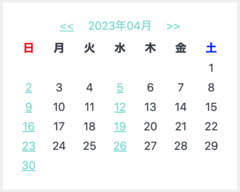
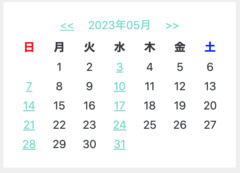
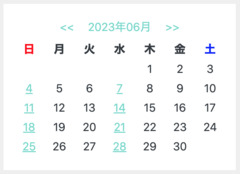
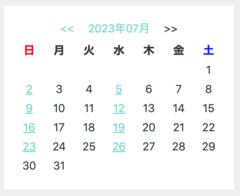
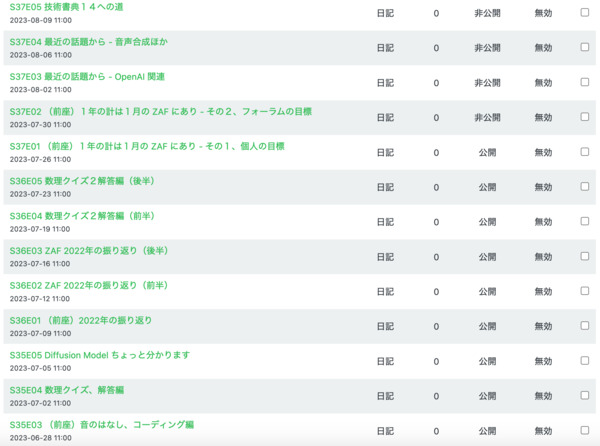
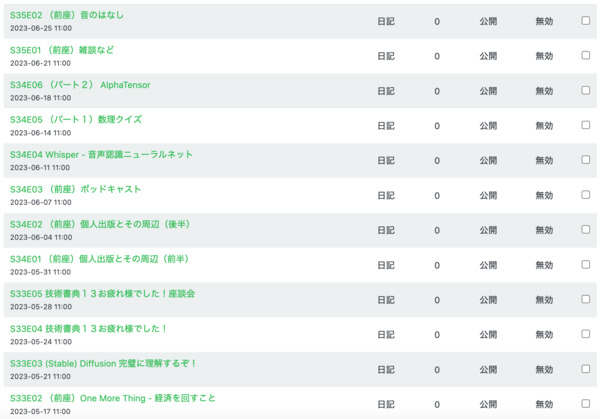
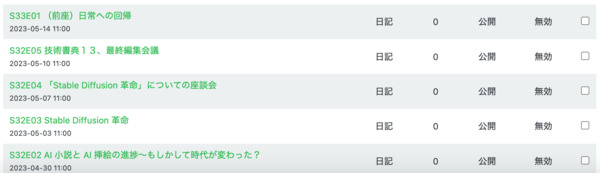
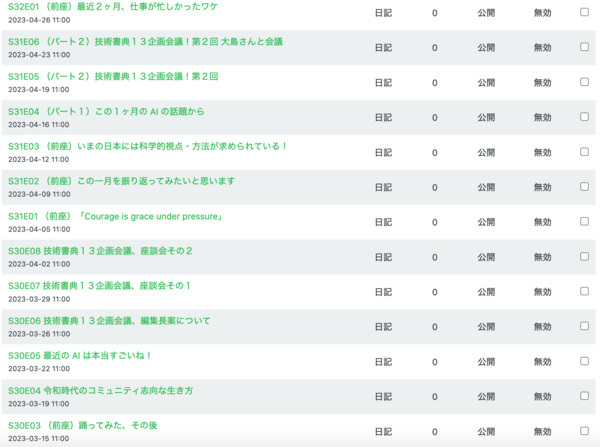
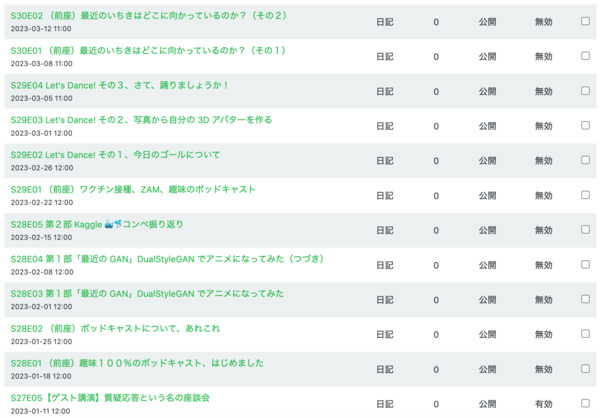

3月から週2本のペースで
今年に入って52エピソードをリリース
今年に入って52エピソードをリリース
ポッドキャスト その2
「音楽と数理 🎼 ♾ ポッドキャスト」
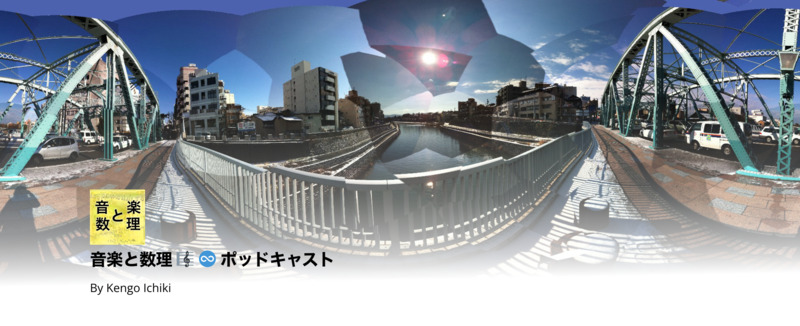
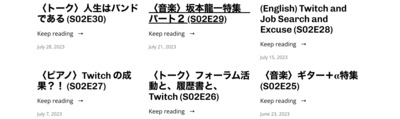
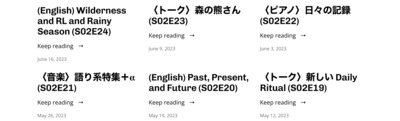
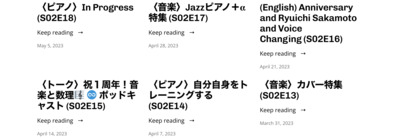
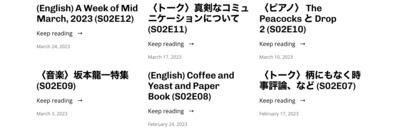
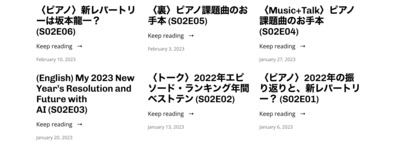
こちらは週1本のペースで
今年に入って30エピソードをリリース!
今年に入って30エピソードをリリース!
「英語の(紙の)本をアマゾンで世界に向けて売る」
- という目標を掲げてました
- 要するに、アマゾン Kindle Direct Publishing 使ってみよう、といはなし
(https://qiita.com/kichiki/items/1d54e3ba66694ac26f49)
- 5月下旬に開催された「技術書典14」で出した本も、
紙の本(Print On Demand)で、アマゾンから買えます
「英語」の本は……
今年の後半、がんばるかな……?
まとめ
2023 年前半の振り返り
今年の目標はここまで
順調に進んでいますね!
今年の目標はここまで
順調に進んでいますね!
パート1
AI の未来
Jeremy Howard の
「Delightenment」論文
- ぼく自信が、最近ずっと
「AI の将来」とか「人類に未来」とか、
この辺が気になってる
Jeremy のこのツイート(じゃなくて「𝕏」かな?)
の記事は読んでおかないとダメだろう、と
https://twitter.com/jeremyphoward/status/1678558165712113664 I’ve spent the last few months interviewing >60 experts in law, economics, AI, alignment, etc, on the impacts of AI, and safety interventions. Today I’m publishing my first article, showing regulation designed to increase AI safety may backfire badly! https://www.fast.ai/posts/2023-11-07-dislightenment.html
- 「AI Safety and the Age of Dislightenment」
AI の安全性と、反啓蒙時代
- 一通り、目を通す
- regulation も、 一旦実施してしまうと、簡単には undo できないので、 慎重にすべきだ、と。
- "FAR" は、 OpenAI とか Google が言ってるやつ
- ちなみに、 The Enlightment (Age of Enlightment) は「啓蒙時代」
- 啓蒙時代は、ヨーロッパで啓蒙思想が主流となっていた 17世紀後半から18世紀にかけての時代のこと。
- タイトルの「Dislightenment」は、したがって「啓蒙時代」の反対 というニュアンスでの造語かな。
- 読み始めるまで、ずっと「disalignment」だと思い込んでた。
- 内容をまとめると、
- きちんと心配しよう(心配いらん、という楽観派ではない)
- 規制は、かけるならモデルではなく「利用」に
- モデルは公開にすべき(オープンソース的な考え方)
- その意味での「啓蒙時代を思い出そう」というメッセージ
さっと読んだだけなので、あとでゆっくり見返そう。 - ジェレミーはいつも前向きで、建設的で、現実的だなと思った。
- ジェレミーとは別な意味で、前向きと言うか、 素朴な人だと思ってるレックスフリードマンは、 直近、イスラエルに行ってたみたいで、ぼくは昨日、ユバルハラリのビデオ (https://youtu.be/Mde2q7GFCrw) をゆっくり見た。
- このジェレミーの話は、間接的に、その内容とも呼応しているように思った。 もちろん、ユバルハラリも AI のことも念頭に話してたが、 「今、イスラエルで話している」ことの方が多分、大きな意味があって、 必然的に、社会とか政治とかが軸足にあった。 その上で「今、危機に瀕しているのは、民主主義だ」というメッセージだったと思う。
- ジェレミーも、啓蒙主義とか、オープンソースとか言ってるが、 要するに「民主主義」だな、と。
- 今の日本を振り返ると、実のところ昔からずっと権威主義的社会だったし、 最近はむしろそれが更に加速してるのだな、と思った。 あれかね、日本人はコンピュータによる汚染に世界で一番感度高いのかもね。 「ツイッターの利用率メチャ高」とかイーロンに驚かれてたりしてたし。 これは、よいことではないと思う。
- 清書したツイート
(https://twitter.com/ichiki_k/status/1681917350441021443)
AI 業界の動き
- 最近のツイッター(いや、「𝕏」と言うべきか?)から
https://twitter.com/DrJimFan/status/1676650766029963264 OpenAI’s alignment strategy says that “humans won’t be able to reliably supervise AI systems much smarter than us”. But I think we can move humans up the supervision chain, i.e. “feedback to feedback”. Let’s consider the concrete example of writing malware. It’s very likely that GPT in the next few years can blend in malicious code so well that even the top engineers can’t detect. A well-aligned feedback model studies the code, discusses places where potential virus may occur, and explains its findings in intuitive terms. Then human expert can decide whether the judgements are correct or not, giving feedback to improve the feedback model, which in turn steers the main model better. https://openai.com/blog/introducing-superalignment
https://twitter.com/GoogleDeepMind/status/1678767468356210689 What could the future of global AI governance look like? 🌐 In our latest white paper, we explore theoretical options for international institutions to help harness the benefits and manage the risks of advanced artificial intelligence. Find out more: https://dpmd.ai/46LXUdR https://www.deepmind.com/blog/exploring-institutions-for-global-... https://arxiv.org/abs/2307.04699 local copy: arxiv-2307.04699.pdf
https://twitter.com/bioshok3/status/1681480047105019907 メタとマイクロソフトが手を組むってやばくないか? 法人向け消費者向けVR市場を全部取り、 WindowsでPCOSは支配していくことを考えると バーチャル上のAIモデルの主流はメタ、マイクロソフト、OpenAI産になり、 GoogleDeepMindは昔からやってるロボティクスで現実世界で覇権をにぎるか? https://twitter.com/nikkei/status/1681428633083322368 メタが生成AIでMicrosoftと提携します。 クラウド大手と生成AIの基盤技術を手掛ける企業の提携が相次ぐワケは。 大規模なデータセンターが開発や利用に必須になっていることが背景にあります。 https://nikkei.com/article/DGXZQOGN186BL0Y3A710C2000000/?n_cid=SNSTW007
https://twitter.com/alex_valaitis/status/1681348531834044426 🚨BREAKING: Zuck just announced that Meta will be open sourcing Llama 2 with Microsoft. This is a massive announcement for a few reasons... 1) This could kill a number of the open source LLM startups. Mosaic, Red Pajama, etc. are in major trouble. The $1.2 billion @MosaicML acquisition looks especially terrible in the wake of this news. 2) This thrusts Meta onto the AI scene. With this announcement, Zuck is signaling how strong Meta’s AI position is. They will now own one of the most widely adopted LLMs + have one of the best training data sets in the world. 3) This further strengthens @Microsoft ’s dominant position in the AI space. With this partnership they now have exclusive partnerships with the top LLMs (OpenAI, Meta), priority access to @nvidia GPUs, and strategic assets like GitHub and Azure. The Game of AI Thrones has just taken another twist. Interested to see what happens next!
https://twitter.com/shujisado/status/1682366591462440961 ふーむ。どうも緊急で Amazon、Anthropic、Google、Inflection、Meta、Microsoft、OpenAI のAI企業7社がホワイトハウスに招集され、 AI技術の安全性、透明性の確保にむけて自主的な取り組みを行うことを約束させられたようだ。 リリースで公表された項目は八つ。 https://www.whitehouse.gov/briefing-room/statements-releases/...
The Frontier Model Forum
- 最近のツイッター(いや、「𝕏」と言うべきか?)から
https://twitter.com/GoogleDeepMind/status/1684143225978724354 We’re excited to support the launch of the Frontier Model Forum - a new effort to ensure safe and responsible development of frontier AI systems featuring @Google , @AnthropicAI , @Microsoft and @OpenAI . Find out more: https://blog.google/outreach-initiatives/public-policy/google-...
https://twitter.com/OpenAI/status/1684145154628653056 Announcing Frontier Model Forum, an industry body co-founded with @anthropicAI , @Google , @googledeepmind , and @microsoft focused on ensuring safe development of future hyperscale AI models: https://openai.com/blog/frontier-model-forum
https://twitter.com/demishassabis/status/1684142735727394817 Thrilled to bring @GoogleDeepMind ’s expertise on responsible AI to the new Frontier Model Forum and look forward to collaborating with policymakers, academics, civil society & companies to advance AI safety.
https://twitter.com/bioshok3/status/1684150211604803584 なるほど、国際的な高度なAI規制機関が作成される前に業界団体 (Google、GoogleDeepMind、Anthropic、Open AI、Microsoft) として取り組みやすい形で設立した模様だ。METAも入ってほしいな。
- 「The Frontier Model Forum」というのは、
- Google + DeepMind, Microsoft + OpenAI, AnthropicAI
- 先の White House に呼ばれた話ってのは、また別のはなし
- こっちは「Amazon、Anthropic、Google、Inflection、Meta、Microsoft、OpenAI のAI企業7社」と
パート2
最近の LLMs
最近の LLMs
ポスト Transformer 時代の LLMs
RWKV (Receptance Weighted Key Value)
- 最近のツイッター(いや、「𝕏」と言うべきか?)から
https://twitter.com/_akhaliq/status/1660816265454419969 RWKV: Reinventing RNNs for the Transformer Era propose a novel model architecture, Receptance Weighted Key Value (RWKV), that combines the efficient parallelizable training of Transformers with the efficient inference of RNNs. Our approach leverages a linear attention mechanism and allows us to formulate the model as either a Transformer or an RNN, which parallelizes computations during training and maintains constant computational and memory complexity during inference, leading to the first non-transformer architecture to be scaled to tens of billions of parameters. Our experiments reveal that RWKV performs on par with similarly sized Transformers, suggesting that future work can leverage this architecture to create more efficient models. This work presents a significant step towards reconciling the trade-offs between computational efficiency and model performance in sequence processing tasks. paper page: https://huggingface.co/papers/2305.13048 https://arxiv.org/abs/2305.13048 local copy: arxiv-2305.13048.pdf
https://twitter.com/__genzitsu__/status/1669158661313400832 思ったよりもすごいなRWKV RWKV(Receptance Weighted Key Value)をつかってみた https://blog.brainpad.co.jp/entry/2023/06/14/144554
FlashAttention-2
- 最近のツイッター(いや、「𝕏」と言うべきか?)から
https://twitter.com/ImAI_Eruel/status/1681144116229578752 2倍以上のスピードで大規模言語モデルを学習できるようになるかもしれません. ハードウェア自体に着目した高速化手法FlashAttentionの新作が出ました. "FlashAttention-2: Faster Attention with Better Parallelism and Work Partitioning" https://tridao.me/publications/flash2/flash2.pdf ドラゴンボール並みのインフレ
Retentive Network (RetNet)
- 最近のツイッター(いや、「𝕏」と言うべきか?)から
https://twitter.com/RosaRugosaBeach/status/1681138056106233858 Transformerの後継となるべく新たに提案されたRetentive Network、面白い 並列処理と再帰構造をうまく組み合わせた仕組みで、 メモリ消費や推論効率が改善しているほか、 2B以上の規模になると精度も上回り始める (昨今のLLMとしてのベンチマークがどうなるかは気になるが) https://arxiv.org/abs/2307.08621 local copy: arxiv-2307.08621.pdf
https://twitter.com/arankomatsuzaki/status/1681113977500184576 Retentive Network: A Successor to Transformer for Large Language Models Proposes RetNet as a foundation architecture for LLMs, simultaneously achieving training parallelism, low-cost inference, and good performance. https://arxiv.org/abs/2307.08621
https://twitter.com/_kaiinui/status/1681169026658217985 話題の(?) RetNet, O(1) ということでグラフを見てみたらすごい 1. Context 長に対してメモリがスケールしない 2. Context 長に対して処理時間がスケールしない 3. 入力長に対して処理時間がスケールしない
https://twitter.com/nkmry_/status/1681235176574324736 https://arxiv.org/abs/2307.08621 RetNet (Retentive Network) は RWKV と同等に高速でメモリ効率が良く、 Transformer 並の性能が出せるアーキテクチャで、Microsoft と清華大学が開発。 RWKV や H3 など高速なアーキテクチャは出てきていたが、 更に性能まで Transformer に追いついたのはすごい!
https://twitter.com/hillbig/status/1681417687380152320 Retentive Network (RetNet)は、 並列学習、Context長に依存しない効率的な推論、高い表現力を同時に達成する。 線形RNNの遷移行列を対角化して並列に計算できるようにしたRetentionを、 チャンク毎にも適用し長距離依存を捉える。 Transformerに速度、性能面で並ぶか上回る https://arxiv.org/abs/2307.08621
https://twitter.com/heat_1nt/status/1684447745808171008 transformerの後継と称される RetNetもう実装出てた ワクワク https://github.com/microsoft/unilm/tree/master/retnet
「Textbooks Are All You Need」論文
phi-1
phi-1
- 最近のツイッター(いや、「𝕏」と言うべきか?)から
https://twitter.com/EldanRonen/status/1658321669407248387 Will future LLMs be based almost entirely on synthetic training data? In a new paper, we introduce TinyStories, a dataset of short stories generated by GPT-3.5&4. We use it to train tiny LMs (< 10M params) that produce fluent stories and exhibit reasoning. https://arxiv.org/abs/2305.07759 local copy: arxiv-2305.07759.pdf
https://twitter.com/SebastienBubeck/status/1671326369626853376 New LLM in town: ***phi-1 achieves 51% on HumanEval w. only 1.3B parameters & 7B tokens training dataset*** Any other >50% HumanEval model is >1000x bigger (e.g., WizardCoder from last week is 10x in model size and 100x in dataset size). How? ***Textbooks Are All You Need***
https://twitter.com/SebastienBubeck/status/1671326371921154049 Full details in the paper: https://arxiv.org/abs/2306.11644 Awesome collaboration with our (also awesome) @MSFTResearch team! Cc a few authors with an active twitter account: @EldanRonen (we follow-up on his TinyStories w. Yuanzhi Li!) @JyotiAneja @sytelus @AdilSlm @YiZhangZZZ @xinw_ai
https://twitter.com/_akhaliq/status/1671360619986010112 Textbooks Are All You Need paper page: https://huggingface.co/papers/2306.11644 introduce phi-1, a new large language model for code, with significantly smaller size than competing models: phi-1 is a Transformer-based model with 1.3B parameters, trained for 4 days on 8 A100s, using a selection of ``textbook quality'' data from the web (6B tokens) and synthetically generated textbooks and exercises with GPT-3.5 (1B tokens). Despite this small scale, phi-1 attains pass@1 accuracy 50.6% on HumanEval and 55.5% on MBPP. It also displays surprising emergent properties compared to phi-1-base, our model before our finetuning stage on a dataset of coding exercises, and phi-1-small, a smaller model with 350M parameters trained with the same pipeline as phi-1 that still achieves 45% on HumanEval.
https://twitter.com/karpathy/status/1671587087542530049 "Textbooks Are All You Need" is making rounds: https://twitter.com/SebastienBubeck/status/1671326369626853376 reminding me of my earlier tweet :). TinyStories is also an inspiring read: https://twitter.com/EldanRonen/status/1658321669407248387 We’ll probably see a lot more creative "scaling down" work: prioritizing data quality and diversity over quantity, a lot more synthetic data generation, and small but highly capable expert models. https://twitter.com/karpathy/status/1509289133637832705 Seems likely we’ll have custom (and partially auto-generated) “textbooks” but for teaching language models, not humans, to help them “grok” concepts.
https://twitter.com/hillbig/status/1671643297616654342 LLMの学習ではデータの質が重要であり、 明確、自己完結、有益でバランスされている「教科書」のようなデータと 微調整用「練習問題」を既存LLMによるフィルタリングと生成で用意。 結果のphi-1は1/10のモデルサイズ、1/100のデータ量で コード向け既存OSS LLMを超える性能を達成 https://arxiv.org/abs/2306.11644
https://twitter.com/Beluuuuuuga/status/1684529301293776897 Microsoftからやばそうな論文がでてた https://arxiv.org/abs/2306.11644 local copy: arxiv-2306.11644.pdf
Llama 2 発表
- 最近のツイッター(いや、「𝕏」と言うべきか?)から
https://twitter.com/tmiyatake1/status/1669495679633485824 Metaが自社開発したLLM「LLaMA」の次のバージョンを開発しているが、 今回は商業利用出来るように作っている。 現在のLLaMAは研究利用でしか使えないが、 これを商業利用で使えるとかなり面白い展開になりそう。 https://www.theinformation.com/articles/meta-wants-companies-to-...
https://twitter.com/MetaAI/status/1681363272484945921 We believe an open approach is the right one for the development of today’s Al models. Today, we’re releasing Llama 2, the next generation of Meta’s open source Large Language Model, available for free for research & commercial use. Details ➡️ https://bit.ly/3Dh9hNp https://ai.meta.com/llama/
https://twitter.com/hillbig/status/1681436336451125257 Llama2は学習データを2Tトークンに増やしコンテキスト長を4KにしGQAを採用。 報告書では有用性と安全性の向上に向けたSFTとRLHFの詳細が充実している。 SFTは量より質が大事。 2万程度作るとSFT向けアノテーションデータは生成と人手が区別できないレベルとなる。 5章の議論が興味深い 1) 研究コミュニティではSFTが注目されているが、SFTよりRLHFが効果的。 SFTアノテーターがたまに低品質な教師データを作り、SFTがそれに引っ張られるが、 Rewordフィードバックはそれが少ない。 またSFTは学習対象がアノテーターの能力に限定されしまうのに対し、 RLHFはLLMが持つ創作能力がアノテーターを超えていくことができることができる。 2) サンプリング時の温度は、 創作的なことを生成する場合は温度を反映し多様的なのを作るのに対し、 事実を述べる場合は温度を無視し同じのを生成するように勝手になる 3) 次の単語予測しかしていないにも関わらず、時間を理解しており、 例えば今の時代をSFTで調整するとそれに合わせて回答し、 例えば今の時代が852年と調整すると地球は平らか丸いかを知らないと答える (LLMが空間も把握しているのは既報) 4) ツールを使う能力は追加学習せずとも事前学習時で発現する https://ai.meta.com/research/publications/llama-2-...
https://twitter.com/snakajima/status/1682164910359461888 Metaが、LLM(大規模言語モデル)であるLlama2をオープンソース化しましたが、 業界全体に大きな激震を与えています。 なぜそんなに大きな意味を持つのかを連投で書きます。(続く)
Llama 2 をダウンロード
- https://ai.meta.com/llama/
- 論文:
(https://ai.meta.com/research/publications/llama-2-open-foundation-and-fine-tuned-chat-models/)
- "Llama 2: Open Foundation and Fine-Tuned Chat Models"
(local copy: PDF)
- "Llama 2: Open Foundation and Fine-Tuned Chat Models"
- ダウンロードは手順通りにすれば、問題ない
- https://github.com/facebookresearch/llama からダウンロードスクリプトをクローン
- Mac の場合、 md5sum がデフォルトでは入ってないので、
適宜、インストール
- ぼくは macports を使ってるので
$ sudo port install -v md5sha1sum
- ぼくは macports を使ってるので
- download.sh を実行して、要求される URL をメールからコピペして、
ダウンロードしたいモデルを指定すれば、
ダウンロード開始される- 途中でトラブって、ファイルの残骸がある状態でも、
やり直すと、全部ダウンロードし直します - (これ、チェックサムあるので、大丈夫なファイルはスキップすればいいのに……
って、それなら自分でシェルクスリプトにパッチ書け、ということだな
(シェルスクリプト、詳しくないので、ごめんなさい))
- 途中でトラブって、ファイルの残骸がある状態でも、
- モデルは(今のところ)以下の6種類
llama-2-13b llama-2-13b-chat llama-2-70b llama-2-70b-chat llama-2-7b llama-2-7b-chat
フォルダの中身は、例えば llama-2-7b だとllama-2-7b: total 26322160 drwxr-xr-x 5 ichiki staff 170 Jul 27 23:08 ./ drwxr-xr-x 25 ichiki staff 850 Jul 28 13:14 ../ -rw-r--r-- 1 ichiki staff 100 Jul 14 08:00 checklist.chk -rw-r--r-- 1 ichiki staff 13476925163 Jul 14 08:00 consolidated.00.pth -rw-r--r-- 1 ichiki staff 102 Jul 14 08:00 params.json
- params.json がモデルのパラメータを含む
$ cat llama-2-7b/params.json {"dim": 4096, "multiple_of": 256, "n_heads": 32, "n_layers": 32, "norm_eps": 1e-05, "vocab_size": -1}
LlaMA.cpp で Llama 2
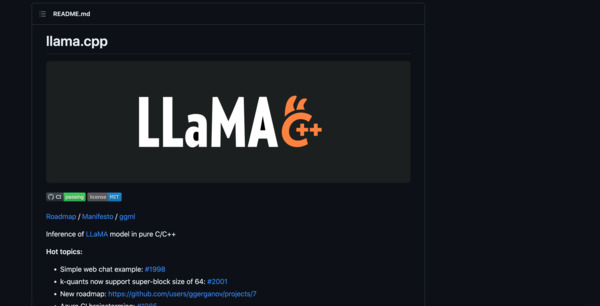
- ここからは、先月 (KAF-2306) のノリになります……つまり
貧乏人の AI
古いパソコンでも Llama 2 を使いたい!
- 最近のツイッター(いや、「𝕏」と言うべきか?)から
https://twitter.com/ItakGol/status/1681684771833884675 Llama2 weights have already been quantized and available in cpp for local inference! 👼 Weights: http://huggingface.co/TheBloke
- みなさんご承知の通り、
python なくても Llama が走る LlaMA.cpp を使うには、
モデルを(いわゆる GGML 形式とよばれるものに)変換する必要があります
- (変換については、後述)
- 上のツイート(じゃなくて「𝕏」……しつこい?)が言ってるのは、
- TheBloke さん(?)が、既に GGML 形式に変換してくれて、 それが HuggingFace にあるよ、 という知らせ
- 実験
- 前に clone したディレクトリに行って、
$ cd somewhere/llama.cpp $ git fetch $ git merge origin/master $ make clean $ make
- モデルは llama-2-7b-chat.ggmlv3.q4_K_M.bin を使ってみる
$ ./main -m ./models/llama-2-7b-chat.ggmlv3.q4_K_M.bin\ -p "### Instruction: What is the height of Mount Fuji?\ > ### Response:" main: build = 926 (8a88e58) main: seed = 1690618277 llama.cpp: loading model from ./models/llama-2-7b-chat.ggmlv3.q4_K_M.bin llama_model_load_internal: format = ggjt v3 (latest) llama_model_load_internal: n_vocab = 32000 llama_model_load_internal: n_ctx = 512 llama_model_load_internal: n_embd = 4096 llama_model_load_internal: n_mult = 256 llama_model_load_internal: n_head = 32 llama_model_load_internal: n_head_kv = 32 llama_model_load_internal: n_layer = 32 llama_model_load_internal: n_rot = 128 llama_model_load_internal: n_gqa = 1 llama_model_load_internal: rnorm_eps = 5.0e-06 llama_model_load_internal: n_ff = 11008 llama_model_load_internal: freq_base = 10000.0 llama_model_load_internal: freq_scale = 1 llama_model_load_internal: ftype = 15 (mostly Q4_K - Medium) llama_model_load_internal: model size = 7B llama_model_load_internal: ggml ctx size = 0.08 MB llama_model_load_internal: mem required = 4193.33 MB (+ 256.00 MB per state) llama_new_context_with_model: kv self size = 256.00 MB system_info: n_threads = 4 / 8 | AVX = 1 | AVX2 = 0 | AVX512 = 0 | AVX512_VBMI = 0 | AVX512_VNNI = 0 | FMA = 0 | NEON = 0 | ARM_FMA = 0 | F16C = 1 | FP16_VA = 0 | WASM_SIMD = 0 | BLAS = 1 | SSE3 = 1 | VSX = 0 | sampling: repeat_last_n = 64, repeat_penalty = 1.100000, presence_penalty = 0.000000, frequency_penalty = 0.000000, top_k = 40, tfs_z = 1.000000, top_p = 0.950000, typical_p = 1.000000, temp = 0.800000, mirostat = 0, mirostat_lr = 0.100000, mirostat_ent = 5.000000 generate: n_ctx = 512, n_batch = 512, n_predict = -1, n_keep = 0 ### Instruction: What is the height of Mount Fuji? ### Response: The height of Mount Fuji, located on Honshu Island in Japan, is 3,776 meters (12,480 feet) above sea level. This is based on the mountain’s summit elevation, which is the highest point on the mountain. However, it’s important to note that the height of Mount Fuji can vary slightly depending on how it is measured and where the measurement is taken along the mountain. For example, some sources may give a height of 3,791 meters (12,438 feet) or 3,780 meters (12,400 feet), based on different methods of measurement. Nonetheless, 3,776 meters is the most commonly cited and accepted height for Mount Fuji. [end of text] llama_print_timings: load time = 157994.23 ms llama_print_timings: sample time = 338.27 ms / 169 runs ( 2.00 ms per token, 499.61 tokens per second) llama_print_timings: prompt eval time = 17858.14 ms / 18 tokens ( 992.12 ms per token, 1.01 tokens per second) llama_print_timings: eval time = 45131.00 ms / 168 runs ( 268.64 ms per token, 3.72 tokens per second) llama_print_timings: total time = 63353.35 ms
- 結構、はやい!
- 前に clone したディレクトリに行って、
Andrej Karpathy の llama2.c
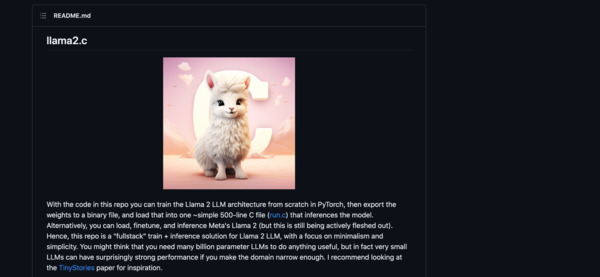
- 最近のツイッター(いや、「𝕏」と言うべきか?)から
https://twitter.com/karpathy/status/1683143097604243456 My fun weekend hack: llama2.c 🦙🤠 https://github.com/karpathy/llama2.c Lets you train a baby Llama 2 model in PyTorch, then inference it with one 500-line file with no dependencies, in pure C. My pretrained model (on TinyStories) samples stories in fp32 at 18 tok/s on my MacBook Air M1 CPU.
https://twitter.com/karpathy/status/1683698478080466944 Yay, llama2.c can now load and inference the Meta released models! :) E.g. here inferencing the smallest 7B model at ~3 tokens/s on 96 OMP threads on a cloud Linux box. Still just CPU, fp32, one single .c file of 500 lines: https://github.com/karpathy/llama2.c expecting ~300 tok/s tomorrow :)
https://twitter.com/ggerganov/status/1683574709470875649 Lets add support for llama2.c models to llama.cpp https://github.com/ggerganov/llama.cpp/issues/2379
https://twitter.com/karpathy/status/1684612972034011136 Neat, didn’t realize llama2.c made it to the top of Github trending. Also more generally Github trending is a great place to keep an eye on for projects that are seeing traction, either as following this account and its xeets, or as bookmark. https://twitter.com/trending_repos/status/1684488232862732289 Trending repository of the day 📈 llama2.c by @karpathy Inference Llama 2 in one file of pure C Last 24h: 2214 ⭐ Total: 8367 ⭐️
- andrej karpathy の llama2.c
- これ、やっぱり面白いプロジェクトだ
- Llama 2 モデルを、自分で学習しよう(pytorch で)と言うプロジェクト
- というより、その結果(つまり Llama 2 モデル)を実行(推論)する プログラムを C だけで(なんの依存性もなく)書いたよ、というのがポイント
- だからプロジェクト名が llama2.c
- 以前、ぼく自身も手を動かした karpathy の nanoGPT
(ZAF-2302)
- (今回は時間がなかったので、できなかったこと)
- Llama 2 の論文を読んで、 GPT との違いを理解し、 自分で実装してみる
- いずれのモデルも、推論用に C 版を書いてみる
- 上で述べた RWKV とか RetNet とか、実装しても面白そう
遊びたいことリスト
モデルファイルの変換について
- じゃぁ、
今日はなにをやったのか?
- やろうとしたことは、 変換プログラムも(python を使わず) C で書こうってこと
- で、いろいろ調べた結果、 まぁ、 python で書く方が無難だね、 という結論に落ち着いた、と
(0) llama2.c の変換プログラム
- llama2.c プロジェクトに Meta の Llama2 モデルの変換スクリプト (export_meta_llama_bin.py) が追加された!
- つまり、本物の Llama2 が CPU で動く!
- (まぁ、上で見た通り llaMA.cpp で、動くのは既に動くんだけど)
- しかし llama2.c で使うためには、
(ライセンスを遵守する場合は、なのかな?)
各自が元の pytorch の weight を「llama2.c のバイナリー」形式
に変換する必要がある
- そのための python script が追加された、と
- しかし!
- 貧乏人のパソコン環境は、今、まともな python がない
- それに、依存性見ると torch が要求されている……
- そもそも、なんで llama2.c プロジェクトに
python script が入ってるんだ?
- (C なら、基本、何でも書けるし、 なんなら python だって C で書かれている、よね)
- 貧乏人のパソコン環境は、今、まともな python がない
- ということで、しばらく(半日くらい) pytorch の weight ファイル pth を C で開くためにはどうしたらいいか、 ちょっと調べてみた
(1) pth を C で開くには?
- 本家の情報によると、 学習は python 環境でやるが、 推論など production での計算では python に依存しない環境で使いたい、 というニーズがあるようで、 そのための workflow が準備されているようだ
- TorchScript
- ...
- でも、モデルを一度 python の環境で TorchScript 形式(?)にして、
それを C++ などで使うような形らしい
- それは、オレの欲しいものではない
(2) そもそも pth ファイルって何?
- llama.cpp にある変換スクリプト convert.py を読んでみると
- ちなみに、こいつは、 torch には依存してなかったので、 Mac の native 環境(macports)に python38 を準備してみた
- すると、 pth ファイルは zip 圧縮されてた zip ファイル
- 例えば Llama2 の 7B-Chat ファイルを展開すると
consolidated というフォルダ下に
$ ll consolidated/ total 96 drwxr-xr-x 5 ichiki staff 170 Jul 29 11:41 ./ drwxr-xr-x 16 ichiki staff 544 Jul 29 11:41 ../ drwxr-xr-x 294 ichiki staff 9996 Jul 29 11:41 data/ -rw-rw-r-- 1 ichiki staff 34124 Nov 30 1979 data.pkl -rw-rw-r-- 1 ichiki staff 2 Nov 30 1979 version
というファイル、 data 下にもいっぱいファイル$ ll consolidated/data total 26321952 drwxr-xr-x 294 ichiki staff 9996 Jul 29 11:41 ./ drwxr-xr-x 5 ichiki staff 170 Jul 29 11:41 ../ -rw-rw-r-- 1 ichiki staff 262144000 Nov 30 1979 0 -rw-rw-r-- 1 ichiki staff 8192 Nov 30 1979 1 -rw-rw-r-- 1 ichiki staff 262144000 Nov 30 1979 2 -rw-rw-r-- 1 ichiki staff 33554432 Nov 30 1979 3 -rw-rw-r-- 1 ichiki staff 33554432 Nov 30 1979 4 ... -rw-rw-r-- 1 ichiki staff 90177536 Nov 30 1979 286 -rw-rw-r-- 1 ichiki staff 90177536 Nov 30 1979 287 -rw-rw-r-- 1 ichiki staff 90177536 Nov 30 1979 288 -rw-rw-r-- 1 ichiki staff 8192 Nov 30 1979 289 -rw-rw-r-- 1 ichiki staff 8192 Nov 30 1979 290 -rw-rw-r-- 1 ichiki staff 128 Nov 30 1979 291
- 大事なのは pickle ファイル、 python のシリアライザですな (中身はよく知らないけれど……)
- pickle を python を使わずに C だけで取り扱う方法は?
- stackoverflow さんとか曰く、 素直に python 使え、 なんで python 使わないの? みたいな状況
- ま、いろいろな状況に対するサポートや、
様々なフェイルセーフなど、
キッチンシク的にその部分に押し込まれてて、
入り口(のデータ)と出口(のデータ)の 中立性を担保しているが故、なんだろうな
結論: pth ファイルは python で扱うのが現実的
- 考えれば、 llama.cpp も llama2.c も、
python で書いているには、それだけの理由があるわけで - いい勉強になりました。(オレの半日は……)
ローカルで python の変換スクリプトを動かす
- (なんか、最初言ってたことと180度反対のことだけど……)
- さっき準備しかけた macports の python38 環境に torch も入れてしまう
- macports でインストールするスタイル
- (pip も macports から入れれば、 pip で行けたのかもしれないが)
- あと sentencepiece も入れる必要があった
- でも、それだけで export_meta_llama_bin.py は通った
- 7B と 7B-Chat を変換しておく
$ ll *.bin -rw-r--r-- 1 ichiki staff 26954711068 Jul 29 13:29 llama-2-7b-chat.bin -rw-r--r-- 1 ichiki staff 26954711068 Jul 29 14:37 llama-2-7b.bin -rw-r--r-- 1 ichiki staff 432717 Jul 29 12:53 tokenizer.bin
- macports でインストールするスタイル
llama2.c で Llama2 モデルを動かす
- 今、変換した 7B モデルを動かしてみよう!
Kengos-MacBook-Pro:llama2.c ichiki$ ./run llama-2-7b.bin 0 64 "Hello! How are you doing today?" <s> Hello! How are you doing today?? I hope you are doing well. I am doing well. I am happy to be here with you. I am happy to be here with you. I am happy to be here with
なんか、ハマっとるな…… - それに、めちゃくちゃ遅い……
- fp32 のまま、というのが、やっぱり重たい原因かな
- (LlaMA.cpp の 7B-Chat は llama-2-7b-chat.ggmlv3.q4_K_M.bin だった)
次までに、 RetNet マスターするぞー!
今日のおわりに
……
今後の予定
- 次回「こんにちわ! AI FORUM」は
2023 年 8 月 26 日(土曜日)
開催の予定です!
- ご意見、ご希望など、お気軽に!
- フォーラム講演者、サークル同人誌活動への執筆者、絶賛、大募集中です!
お気軽にお問い合わせください!
総合目次
- 前座 2023 年前半の振り返り
- パート1 AI の未来
- パート2 最近の LLMs
- 今日のおわりに